How do you measure and track FCR, or First Call Resolution Rate?
First Call Resolution (FCR) is a key indicator of call center efficiency and customer satisfaction. It measures the ability to resolve customer issues on first contact, reducing the need for follow-ups and improving the customer experience.
In this article, we'll explain how to calculate this rate, the different measurement methods available, and the advantages and disadvantages of each.
Why track first call resolution rates in contact centers?
FCR is a relevant KPI that can have benefits on all other key performance indicators such as customer satisfaction, costs and sales.
According to SQM, for every 1% improvement in first-contact resolution, this results in :
- CSAT improved by +1%.
- Operating costs down -1%.
- Improved employee satisfaction by +1 to +5%.
According to the same study, when a situation is resolved, the customer conversion rate increases by +20%, and 98% of customers will remain loyal to the brand if the situation is resolved at first contact.
This virtuous circle of customer satisfaction makes it clear that the FCR metric is interconnected with other essential customer relationship center metrics such as CSAT, ESAT, costs and sales.
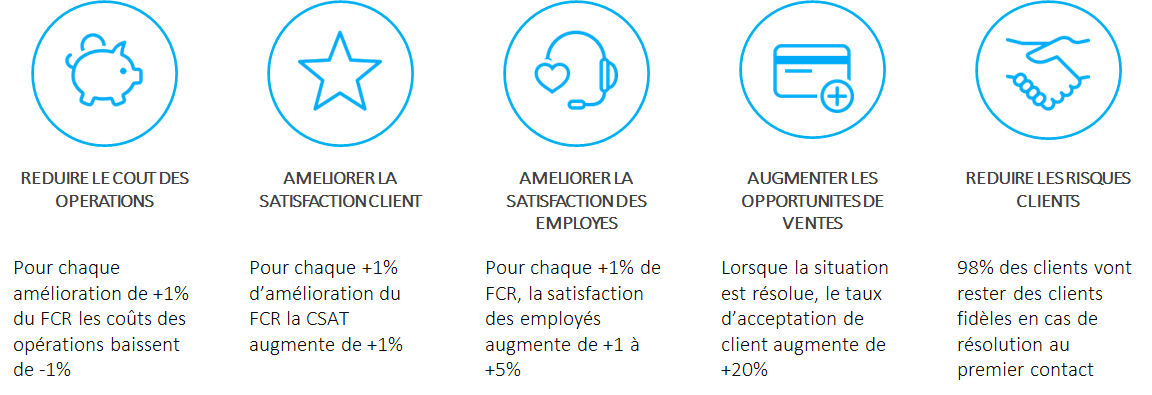
How to calculate the RCF?
The first call resolution rate is calculated by dividing the number of requests resolved on the first call by the total number of calls. Then multiply the result by 100 to obtain a percentage.
For example, if a call center receives 200 calls and manages to solve 150 problems on the first call, the calculation is as follows:
150 / 200 * 100 = 75%
A good FCR score is between 70 and 80%.

Measure first-call resolution using external data
By "external data" we mean data based on direct feedback from customers to assess problem resolution from the very first contact.
We agree that this is the best source of information for accurately measuring FCR and evaluating the quality of the customer experience.
But the response rate is variable, which can limit the representativeness of the data. In addition, customers may be influenced by emotional or contextual factors when responding, which can affect objectivity.
Here are the main ways to gather customer feedback:
Post-call telephone survey
Post-call telephone surveys involve contacting customers by telephone after their initial interaction to ask them whether they feel their problem was resolved during the first call. This method provides direct and immediate feedback on the effectiveness of the resolution.
Advantages :
- The customer determines the RCF/resolution of his request
- High reliability
- Identifies dissatisfied customers
Disadvantages :
- High cost
- Small sample due to customers' willingness to respond
- Processing time >1 day after call
Post-call e-mail survey
Post-call e-mail surveys involve sending an e-mail to customers after their initial interaction, asking them to answer questions to assess whether their problem was solved on first contact. This method enables detailed feedback to be collected asynchronously.
Advantages :
- The customer determines the FCR / resolution of his request
- Low cost
- Identifies dissatisfied customers
Disadvantages :
- Average cost (treatment-related)
- Reduced sample due to customers' willingness to respond and availability of e-mail address
- Limited feedback (reduced number of questions to maximize responses)
- Processing time >1 day after call
Outgoing post-call IVR (Interactive Voice Response) survey
The post-call outbound IVR survey uses an Interactive Voice Response (IVR) system to call customers after their initial interaction and ask them automated questions about their problem resolution.
This method makes it possible to collect feedback quickly and automatically.
Advantages :
- The customer determines the FCR / resolution of his request (reliable unit declaration)
- Identifies dissatisfied customers (can be linked to CSAT)
- Low cost (automated process)
- Rapid availability of information
Disadvantages :
- Small sample due to customers' willingness to respond
- Customers rarely appreciate contact with a robot
Inbound post-call IVR (Interactive Voice Response) survey
The inbound post-call IVR survey invites customers to complete a questionnaire via an interactive voice response (IVR) system immediately after their initial interaction, usually by staying on line or calling a specific number.
This method provides instant, automated feedback on problem resolution.
Advantages :
- The customer determines the FCR the resolution of his request (reliable unit declaration)
- Identifies dissatisfied customers (can be linked to CSAT)
- Low cost (automated process)
- Rapid availability of information
Disadvantages :
- Unreliable and sampled
- Can be manipulated (proposal by advisor only in case of successful calls)
- Limited feedback (reduced number of questions to maximize responses)
- Customers rarely appreciate contact with a robot
Inquiry by advisor during call
Inquiry by the advisor during the call consists of the advisor asking the customer directly, before concluding the call, whether he feels his problem has been solved.
This method provides immediate, direct feedback on the quality of the resolution.
Advantages :
- Cost diluted in the customer relations process
- Involves the advisor in RCF monitoring
- Can be linked to the Quality Management (QM) process
Disadvantages :
- Consultants don't like scripted questions
- The survey can be detrimental to the overall experience
- Can be manipulated (proposal by advisor only in case of successful calls)
- Customers may not dare to be sincere
- Limited feedback (the number of questions is reduced to maximize responses)
Measure First Call Resolution using internal data
Measuring CRF from internal data involves using information gathered via internal software and systems. These methods rely on the analysis of interactions and call data to assess problem resolution.
These methods have the advantage of being more objective, as they are less subject to customers' emotional biases. What's more, this data can be collected continuously and in real time, offering a constant view of performance.
But internal data may not fully reflect customer satisfaction or perception. And analyzing this data can be complex, requiring sophisticated tools and advanced analytical skills.
Here are the main methods used to analyze the FCR with internal data:
Automatic detection of multiple calls
Automatic multiple call detection uses software to identify customers who contact customer service several times for the same problem.
Advantages :
- Automated process
- Enables a quantitative approach
- Selects calls to listen to for a targeted QM approach
- Based on significant data volumes
Disadvantages :
- Based on deterministic and subjective resolution rules
- May differ from actual RCF rate (+/- 10 to 20%)
- Limited feedback (no qualitative information)
Quality Management
Quality Management involves the evaluation of interactions between agents and customers by supervisors or automated tools such as Batvoice, to check whether problems have been correctly resolved on the first call.
This method ensures high quality standards and identifies areas for improvement.
Advantages :
- Based on existing quality management processes
- Provides coaching for advisors on calls listened to
- Costs diluted in the quality process
Disadvantages :
- High cost (for listening and processing)
- Reduced sample size Based on deterministic and subjective resolution rules (evaluator)
- May underestimate or exaggerate actual RCF rate (+/- 10 to 20%)
- Can be intrusive for advisors
CRM and ticket management
This method involves using a Customer Relationship Management (CRM) system to track and analyze support tickets. It allows you to determine whether customer problems are being resolved at the first contact by checking ticket history and updates.
Advantages :
- Based on existing systems
- Enables a quantitative approach
- Low costs (check box in drop-down menu)
- Identifies unresolved queries
Disadvantages :
- Based on deterministic and subjective resolution rules
- Can be distorted by advisors (subjective statement)
- May differ from actual RCF rate
- Limited feedback (little qualitative information in self-evaluation)
Which method do you choose to measure your customer service CRF?

To manage the activities of your CRC (customer relationship center), it's essential to select one or more measurement methods in order to get a complete picture of your performance and balance the advantages and disadvantages.
The question is how to reconcile :
- Data completeness and reliability for a statistical approach
- Qualitative content (customer feedback) to identify priority areas for improvement
- Automation to streamline implementation without changing processes
- Return on investment
The best of both worlds: Speech Analytics and automatic Quality Management
Artificial intelligence provides some answers, with Speech Analytics applied to the voice of the customer and Quality Management processes. This technology involves analyzing all calls to a contact center and extracting both quantitative and qualitative data.
Advantages :
- Completeness of analysis (all calls recorded)
- Instant and continuous call processing
- High reliability
- Identify dissatisfied customers (irritants, strong anger) and associate them with automated actions
- Allows you to obtain spontaneous feedback from customers without additional steps, thanks to Voice of Customer
- Allows you toidentify areas for improvement and monitor progress on an ongoing basis (quality management).
- Automates the identification of re-calls and reasons for re-calls
Disadvantages :
- Involve teams around the Voice of the Customer (VOC) to launch actions on cross-functional company processes.
- Additional costs may be incurred for consultancy support.
- Analysis models are sometimes generic (depending on the specific features of Speech Analytics solutions) and may lack precision depending on the business sector.
- ROI is difficult for companies to measure, and requires expertise from the Speech Analytics provider
- Generic AI models are sometimes proposed: these are less efficient than specific models (specific calibrated AIs require more effort and cooperation for optimal performance).